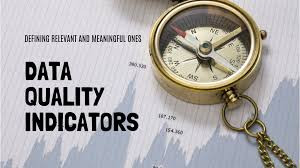
Organizations are continuously inundated with information from multiple sources. This deluge of data presents both opportunities and challenges. On one hand, high-quality data can empower decision-makers, enhance operational efficiencies, and drive strategic initiatives. On the other hand, poor data quality can lead to misguided strategies, wasted resources, and lost revenue opportunities. As organizations strive to harness the power of data, data quality indicators become essential tools for assessing and improving organizational intelligence.Understanding Data Quality Indicators
Data quality indicators are metrics designed to evaluate the accuracy, completeness, consistency, timeliness, and relevance of data. By measuring these attributes, organizations can gain insights into the health of their data and identify areas for improvement. Various frameworks exist to define and categorize these indicators, often focusing on dimensions such as validity, reliability, and accessibility. For effective decision-making, it’s crucial for organizations to adopt a systematic approach to viewing data quality indicators and integrate them into their data management practices.The Dimensions of Data Quality
To effectively assess data quality, organizations can explore several key dimensions. 1. Accuracy: This dimension assesses whether the data correctly represents the real-world constructs it is intended to measure. Accurate data reflects true values and minimizes errors, which is critical for decision-making.2. Completeness: Completeness measures whether all required data is present. Missing data can skew analyses and lead to faulty conclusions. Organizations must ensure they capture all necessary information to maintain a holistic view.3. Consistency: Consistency involves ensuring that data remains stable and coherent across different systems and datasets. Conflicting information can undermine trust in data and lead to confusion.4. Timeliness: Timeliness evaluates the age of the data and its relevance to current decisions. Stale data can result in outdated insights, which can be particularly detrimental in rapidly changing environments.5. Relevance: Relevance assesses whether the data meets the needs of the organization and its stakeholders. Data must be pertinent to the specific context and objectives of the analysis being conducted.These dimensions serve as the foundation for developing data quality indicators that can be tailored to meet the unique needs of an organization.Implementing a Data Quality Framework
To effectively leverage data quality indicators, organizations should implement a data quality framework. This framework should include the following components:1. Data Governance: Establishing clear data governance policies ensures accountability for data management and quality. Appointing data stewards or a data governance committee can help oversee data quality initiatives and foster a culture of data stewardship across the organization.2. Data Profiling: This involves assessing existing datasets to understand their structure, content, and quality. By profiling data, organizations can identify anomalies, inconsistencies, and gaps that need to be addressed.3. Quality Assessment: Regularly conducting quality assessments based on defined indicators enables organizations to track improvements over time. These assessments should involve both automated tools and manual reviews to ensure a comprehensive evaluation.4. Continuous Improvement: Data quality is not a one-time initiative, but an ongoing process. Organizations should establish feedback loops that enable continuous monitoring and enhancement of data quality practices. This could involve training staff, adopting new technologies, or refining data collection processes.The Role of Technology in Data Quality Management
In an era of big data and advanced analytics, technology plays a crucial role in managing data quality. Organizations can utilize various data management tools and software solutions to automate data quality checks and streamline processes. Data cleansing tools can help identify and rectify inaccuracies, while data integration platforms can ensure consistency across disparate systems.Moreover, machine learning algorithms can be employed to predict data quality issues based on historical trends. By analyzing patterns, organizations can proactively address potential problems before they impact decision-making.The Business Impact of High Data Quality
Investing in data quality yields significant business benefits. Organizations that prioritize data quality are better equipped to make informed decisions, respond to market changes, and innovate. High-quality data enables accurate forecasting, effective targeting of customers, and improved risk management.Furthermore, maintaining data quality can enhance customer trust and satisfaction. When organizations provide reliable information, customers feel more confident in their decisions, fostering loyalty and engagement. Ultimately, high data quality can result in a competitive advantage, positioning organizations for success in their respective industries.Challenges in Data Quality Management
Despite its importance, managing data quality presents several challenges. One major hurdle is the sheer volume and variety of data that organizations encounter. With data coming from various sources—such as social media, IoT devices, and customer interactions—ensuring consistent quality across all datasets can be daunting.Additionally, the evolving nature of data regulations and compliance requirements adds complexity to data quality management. Organizations must remain vigilant in adapting their practices to align with legal standards while also maintaining data integrity.Engaging Stakeholders in Data Quality EffortsFor data quality initiatives to be successful, they must involve various stakeholders across the organization. Engaging departments such as IT, operations, marketing, and finance ensures a collaborative approach to data management. Cross-functional teams can provide diverse perspectives on data use and help identify quality issues that may go unnoticed in siloed environments.Training and raising awareness about the significance of data quality among employees is also crucial. When staff members understand the impact of their contributions to data quality, they are more likely to prioritize accuracy and completeness in their work.Data quality indicators serve as a strategic lens through which organizations can assess and improve their organizational intelligence. By focusing on the dimensions of data quality and implementing a robust framework for management, organizations can enhance decision-making, operational efficiency, and customer satisfaction. In an increasingly competitive landscape, leveraging high-quality data is not just an operational necessity; it is a strategic imperative that can drive sustained success.